Why you're doing ad hoc analytics wrong
Before your next SQL dumpster dive: ask why and document everything
When I was a data scientist at Airbnb, the most common question I got was:
“Do you know how many people clicked on this button?”
But the curious thing about this question: it was rarely the question that the stakeholder actually wanted answered.
Ad hoc questions like this are an unavoidable (and substantial!) part of data science/analytics work, yet this type of work is seldom discussed, let alone optimized or reflected on. We as analysts have developed impressive technical competencies to skillfully answer these kinds of questions, but few of us have learned to maximize the impact of our answers — to get to the root of the question and answer that instead. To wit: we‘re good at answering questions, but we’re not good at finding the right questions to answer.
Some introspection here can help shape whether your team operates as help desk SQL monkeys or as strategic partners in stakeholder collaborations. In what follows, I’ll discuss two practical ways to elevate ad hoc work in service of the latter: ask why and document everything.
Step 1: Always Ask Why
The first and most important step when starting ad hoc work is to simply ask why the request needs to be done. What’s the business objective? What question is being answered? Questions asked of analysts are too often red herrings. But behind every quick question is usually a valuable strategic request. Simply asking why before dumpster diving into the data can help uncover the true, strategic question hidden underneath. This is critical for there reasons:
Better decisions
Asking why always leads to better decisions. If you understand the business objective, you’re able to find data that directly addresses it, rather than relying on a stakeholder’s attempt to do so. Non-technical folks are simply not great at knowing what data they need (nor should they be expected to be), let alone what analyses are even possible. The onus, therefore, is on us to ensure that the data we pull is the data they deserve, not the data they “need” (cue Dark Knight soundtrack).More interesting work
Asking why leads to substantially more interesting questions for us. For instance, “how do we measure impact without an experiment” is far more interesting than the button-click question I started at the beginning of the article. I suspect many of us understand that asking why would be the preferred way to respond, but we intentionally ignore that noble inner voice of ours to protect our time for our own, more intellectually satisfying projects. But this is the wrong mindset.Uncover better projects for yourself
Answer enough strategic requests, and you’ll find there are often patterns to be had underneath. These can lead to the sorts of longer-term projects that analytics teams should be working on. We ought to be running our data teams like product teams, which happens by solving our stakeholder’s problems, not by building another unused trashboard. “A user-centered focus is key”, and ad hoc work is the primary way we become user-centered.
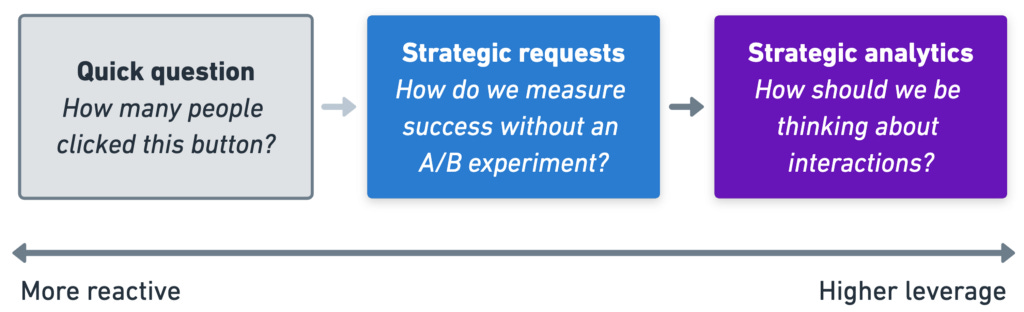
Step 2: Document. Everything.
Once you’ve asked why, the next highest-leverage step you can take is to actually write down what you’ve done. Write down:
The business objective (the one you just aligned on)
Your approach
Your findings (along with your SQL queries, to be able to reproduce this work)
Any decisions that were based on your work.
Put it in a place that’s discoverable by your teammates, and create templates so cognitive load is minimized when skimming through past work. Tools can make a huge difference here. At Airbnb, we tried to use Github and Google docs for this purpose, but the problem with these efforts is that the always fell to the wayside in high urgency situations. Modern document workspaces work substantially better — they’re searchable, easy to write in, and seamless for collaboration.
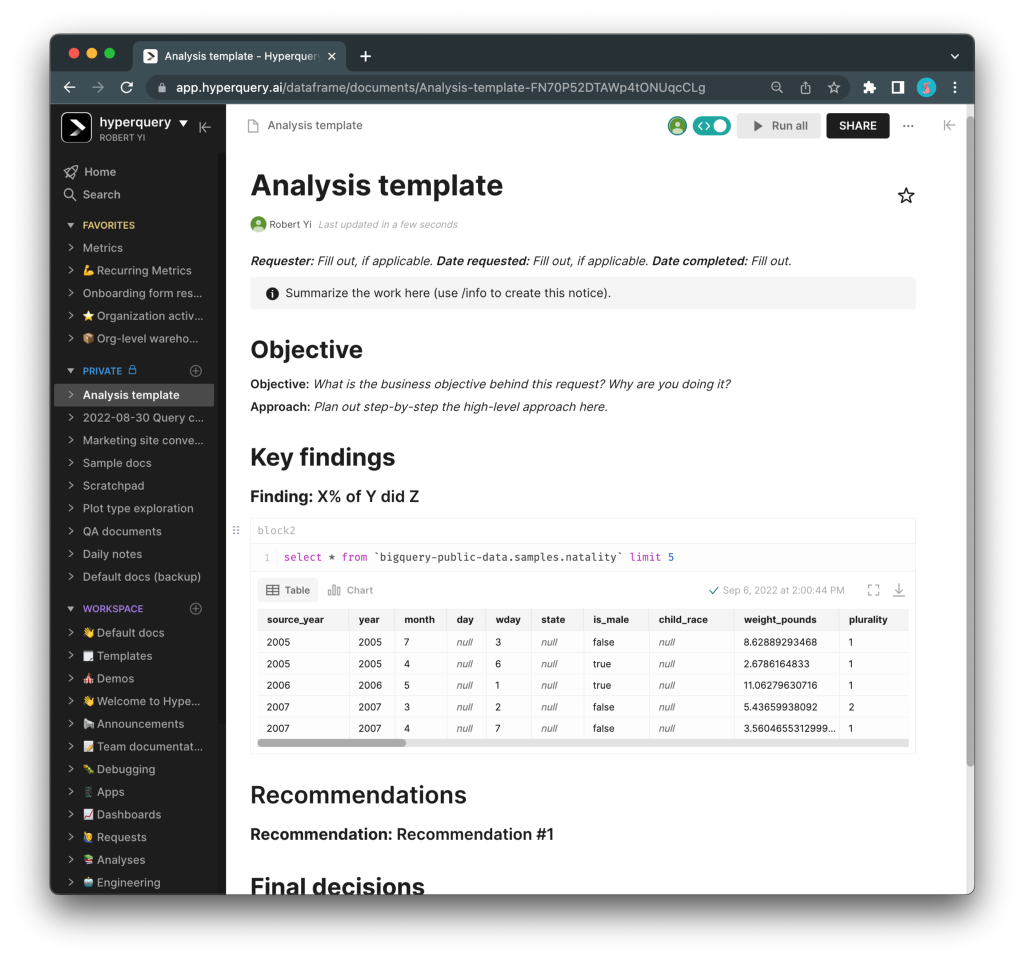
It’s easy to dismiss this part of the workflow as soon as you feel “done” with an analysis. But documenting helps both you and others leverage it beyond the single decision, giving your work scale. Your stakeholders will benefit from being able to revisit their decisions and the precise justifications for them, but you and your fellow analysts can search through your library of work when similar questions inevitably arise or, better, find patterns that can inspire future high-leverage endeavors.
Final comments
Ad hoc work comprises 40–50% of analyst time, but the amount of mindshare devoted to it is rarely commensurate. By consistently asking why and implementing some basic documentation practices, you can make this time not only more impactful, but more rewarding as well. That said, regardless of the finer operational points, establishing some sort of strategy or set of best practices for your team to elevate ad hoc work is certainly worth your while.
If you have any strategies you’ve had success with yourself, let us know!
Interested in learning more about super-powering your ad hoc workflows? Reach out to me at robert@hyperquery.ai, and check out our dedicated platform for ad hoc analytics at hyperquery.ai.
Why You’re Doing Ad Hoc Analytics Wrong was originally published in Towards Data Science on Medium, where people are continuing the conversation by highlighting and responding to this story.